Convolutional neural networks (CNNs) are very important deep neural networks for analyzing visual imagery. However, most CNN-based methods have the problem of over-smoothing at boundaries, which is unfavorable for hyperspectral image classification. To address this problem, a spectral-spatial multiscale residual network (SSMRN) by fusing two separate deep spectral features and deep spatial features is proposed to significantly reduce over-smoothing and effectively learn the features of objects. In the implementation of the SSMRN, a multiscale residual convolutional neural network is proposed as a spatial feature extractor and a band grouping-based bi-directional gated recurrent unit is utilized as a spectral feature extractor. Considering that the importance of spectral and spatial features may vary depending on the spatial resolution of images, we combine both features with two weighting factors with different initial values that can be adaptively adjusted during the network training. To evaluate the effectiveness of the SSMRN, extensive experiments are conducted on public benchmark data sets. The proposed method can retain the detailed boundary of different objects and yield competitive results compared with several state-of-the-art methods. |
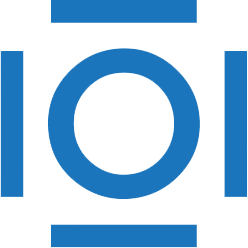
CITATIONS
Cited by 2 scholarly publications.
Image classification
Education and training
Feature extraction
Hyperspectral imaging
Spatial resolution
Data modeling
Neural networks