Developing an efficient method to accurately estimate aboveground biomass in tropical forests is critical to monitoring the carbon stock and implementing policies to reduce emissions caused by deforestation. Thus, the objective of the present study was to estimate aboveground biomass in areas of the Amazonian Forest with selective logging, using the random forest (RF), support vector machine (SVM), and artificial neural network (ANN) machine learning algorithms, using light detection and ranging (LiDAR) data and these combined with OLI/Landsat 8 variables, as well as mapping the biomass for the years 2014 and 2017, allowing one to analyze its dynamics between the years of analysis. The RF and SVM algorithms obtained the lowest error values in all datasets. The association of the variables increased the RF performance. Analyzing the dynamics of biomass, it was observed that the oldest exploration units (2006, 2007, and 2008) have lower biomass stocks. The highest biomass losses in 2017 came from units operated between 2012 and 2013 (the most recent record). Thus, with the method used in this study, it was possible to infer that the machine learning algorithms were efficient in estimating the biomass, emphasizing the RF and the SVM. |
ACCESS THE FULL ARTICLE
No SPIE Account? Create one
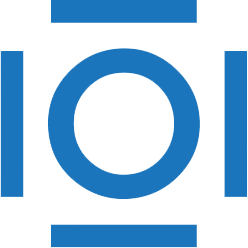
CITATIONS
Cited by 2 scholarly publications.
LIDAR
Biomass
Biological research
Data modeling
Vegetation
Machine learning
Rain