|
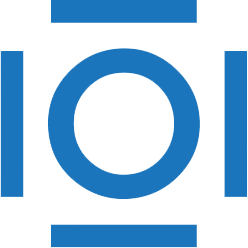
CITATIONS
Cited by 29 scholarly publications.
Optical coherence tomography
Speckle
Signal to noise ratio
Digital filtering
3D image processing
Detection and tracking algorithms
Image enhancement