Significance: Morphological changes in the epidermis layer are critical for the diagnosis and assessment of various skin diseases. Due to its noninvasiveness, optical coherence tomography (OCT) is a good candidate for observing microstructural changes in skin. Convolutional neural network (CNN) has been successfully used for automated segmentation of the skin layers of OCT images to provide an objective evaluation of skin disorders. Such method is reliable, provided that a large amount of labeled data is available, which is very time-consuming and tedious. The scarcity of patient data also puts another layer of difficulty to make the model more generalizable. Aim: We developed a semisupervised representation learning method to provide data augmentations. Approach: We used rodent models to train neural networks for accurate segmentation of clinical data. Result: The learning quality is maintained with only one OCT labeled image per volume that is acquired from patients. Data augmentation introduces a semantically meaningful variance, allowing for better generalization. Our experiments demonstrate the proposed method can achieve accurate segmentation and thickness measurement of the epidermis. Conclusion: This is the first report of semisupervised representative learning applied to OCT images from clinical data by making full use of the data acquired from rodent models. The proposed method promises to aid in the clinical assessment and treatment planning of skin diseases. |
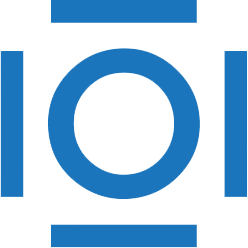
CITATIONS
Cited by 1 scholarly publication.
Data modeling
Image segmentation
Optical coherence tomography
Skin
Human subjects
Tumor growth modeling
Combustion