|
ACCESS THE FULL ARTICLE
No SPIE Account? Create one
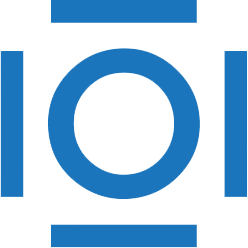
CITATIONS
Cited by 4 scholarly publications.
Image segmentation
Visualization
Remote sensing
Data modeling
Visual process modeling
Medical imaging
Associative arrays