|
ACCESS THE FULL ARTICLE
No SPIE Account? Create one
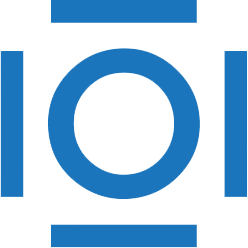
CITATIONS
Cited by 27 scholarly publications.
Image classification
Light emitting diodes
Light sources and illumination
Databases
RGB color model
Visualization
Feature extraction