|
ACCESS THE FULL ARTICLE
No SPIE Account? Create one
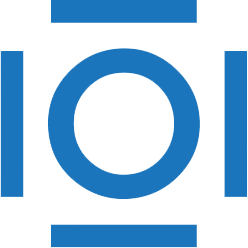
CITATIONS
Cited by 2 scholarly publications.
Hyperspectral imaging
Solid state lighting
Image classification
Data analysis
Associative arrays
Alternate lighting of surfaces
Machine learning