|
ACCESS THE FULL ARTICLE
No SPIE Account? Create one
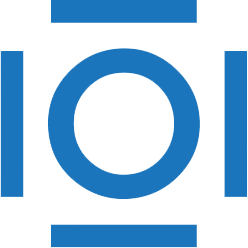
CITATIONS
Cited by 3 scholarly publications.
Convolution
Image denoising
Denoising
Performance modeling
Data modeling
Deconvolution
Network architectures