Action recognition has wide applications in fields such as human–computer interaction, virtual reality, and robotics. Since human actions can be represented as a sequence of skeleton graphs, approaches based on graph neural networks (GNNs) have attracted considerable attention in the research action recognition. Recent studies have demonstrated the effectiveness of two-stream GNNs in which discriminative features for action recognition are extracted from both the joint stream and the bone stream. Each stream is generated by GNNs that support message passing along fixed connections between vertices. However, existing two-stream approaches have two limitations: no interaction is allowed between the two streams and temporary contacts between joints or bones cannot be modeled. To address these issues, we propose the interactive two-stream graph neural network, which employs a joint–bone communication block to accelerate the interaction between the joint stream and the bone stream. Furthermore, an adaptive strategy is introduced to enable dynamic connections between vertices. Extensive experiments on three large-scale datasets have demonstrated the effectiveness of our proposed method. |
ACCESS THE FULL ARTICLE
No SPIE Account? Create one
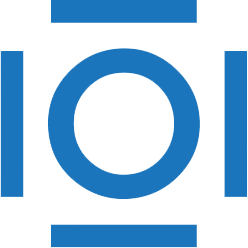
CITATIONS
Cited by 1 scholarly publication.
Bone
Neural networks
Data modeling
RGB color model
Convolution
3D modeling
Video