Generative adversarial network (GAN) has made great progress in image generation and reconstruction, but it may cause the mode collapse problem in practice. We proposed the dual discriminator weighted generative adversarial network (D2WGAN), whose objective function weights the Kullback–Leibler divergence (KL divergence) and the reverse KL divergence and uses the complementary characteristics of these two divergences to make the generated models more diverse. Moreover, we proved the theoretical conditional optimality of the D2WGAN to show that the generator can learn the real data distribution. Finally, we conduct experiments on a large amount of synthetic data and real-world datasets (e.g., MNIST and CIFAR-10). The results show that, compared with the traditional dual discriminator generative adversarial network and GAN, the proposed D2WGAN can process multiple mode data and generate better sample diversities. |
ACCESS THE FULL ARTICLE
No SPIE Account? Create one
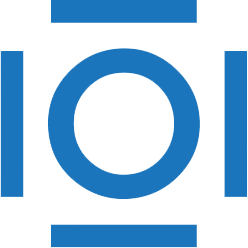
CITATIONS
Cited by 2 scholarly publications.
Gallium nitride
Data modeling
Image quality
Reverse modeling
Data centers
Image enhancement
Optimization (mathematics)