We present an image inpainting technique using frequency-domain information. Prior works on image inpainting predict the missing pixels by training neural networks using only the spatial-domain information. However, these methods still struggle to reconstruct high-frequency details for real complex scenes, leading to a discrepancy in color, boundary artifacts, distorted patterns, and blurry textures. To alleviate these problems, we investigate if it is possible to obtain better performance by training the networks using frequency-domain information (discrete Fourier transform) along with the spatial-domain information. To this end, we propose a frequency-based deconvolution module that enables the network to learn the global context while selectively reconstructing the high-frequency components. We evaluate our proposed method on the publicly available datasets: celebFaces attribute (CelebA) dataset, Paris streetview, and describable textures dataset and show that our method outperforms current state-of-the-art image inpainting techniques both qualitatively and quantitatively. |
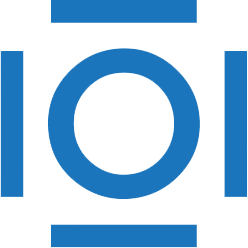
CITATIONS
Cited by 8 scholarly publications.
Deconvolution
Convolution
Visualization
Image segmentation
Image quality
Data modeling
Network architectures