Thangka Yidam classification is of great importance for understanding the image content and experiencing the Tibetan living culture. However, few samples, complex composition, and diverse Thangka images give the present methods a challenge for Thangka Yidam classification. To this end, we propose an improved method for Thangka Yidam classification based on DenseNet and SENet, which improves the transmission and reuse of feature information. First, a weighted pooling function is designed in the squeeze operation of SENet to alleviate the loss of feature information. Second, we further embed the improved SENet between dense blocks and transition layers of DenseNet to learn the weight correlation between feature channels. Finally, we adopt the Leaky ReLU function to optimize the network in DenseNet. The experimental results demonstrate that the accuracy of our method on the constructed Thangka dataset is increased by 5.54% to 95.89% compared with DenseNet model, and the accuracy on the Cifar-10 dataset is increased by 2.23% to 92.46%. Moreover, the experiments on Thangka dataset also show the significant improvements of our approach over the state-of-the-art methods, which proves the generalization ability of our method. |
ACCESS THE FULL ARTICLE
No SPIE Account? Create one
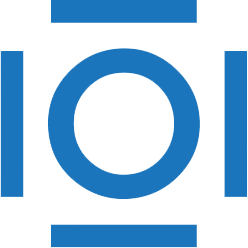
CITATIONS
Cited by 3 scholarly publications.
Image classification
Convolution
Data modeling
Feature extraction
Image segmentation
Performance modeling
Image understanding