Jointly learned detectors and descriptors are becoming increasingly popular because they can simplify the matching process and obtain more correspondences than traditional tools. However, most methods yield low keypoint detection accuracy due to the large receptive field of the detection score map. In addition, existing methods lack efficient detector loss functions because the coordinates of keypoints are discrete and nonderivable. To mitigate these two problems, we propose a method called dynamic attention-based detector and descriptor with effective and derivable loss (DA-Net). For the first problem, a dynamic attention convolution-based feature extraction module is proposed to select the most suitable parameters for different samples. In addition, a multilayer feature self-difference detection (MFSD) module is proposed to detect keypoints with high accuracy. In the MFSD module, multilayer feature maps are used to calculate their feature self-difference maps, and they are fused to obtain a detection score map. For the second problem, an approximate keypoint distance loss function is proposed by approximately regressing the coordinates of the local maximum as keypoint coordinates, allowing the calculations involving keypoint coordinates to backpropagate. Moreover, two descriptor loss functions are proposed to learn reliable descriptors. A series of experiments based on widely used datasets show that DA-Net outperforms other learned detection and description methods. |
ACCESS THE FULL ARTICLE
No SPIE Account? Create one
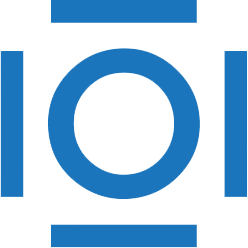
CITATIONS
Cited by 1 scholarly publication.
Education and training
Convolution
Feature extraction
Visualization
3D modeling
Ablation
3D image reconstruction