|
ACCESS THE FULL ARTICLE
No SPIE Account? Create one
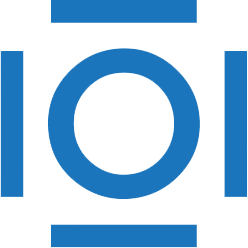
CITATIONS
Cited by 17 scholarly publications.
Sensors
Neural networks
Statistical analysis
Error analysis
Optical engineering
Computing systems
Data fusion