|
ACCESS THE FULL ARTICLE
No SPIE Account? Create one
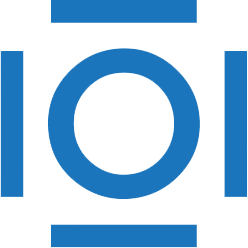
CITATIONS
Cited by 9 scholarly publications.
Image segmentation
Image processing algorithms and systems
Optical engineering
Speckle
Binary data
Reflectivity
Ultrasonography