|
ACCESS THE FULL ARTICLE
No SPIE Account? Create one
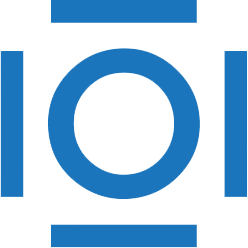
CITATIONS
Cited by 1 scholarly publication.
Cameras
RGB color model
Neural networks
Solid modeling
Computer aided design
Convolutional neural networks
Image processing