|
ACCESS THE FULL ARTICLE
No SPIE Account? Create one
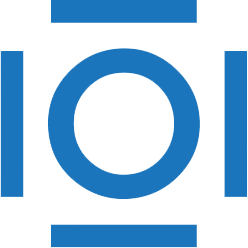
CITATIONS
Cited by 6 scholarly publications.
Wavelets
Neural networks
Quantization
Image compression
Convolution
Mammography
Linear filtering