|
ACCESS THE FULL ARTICLE
No SPIE Account? Create one
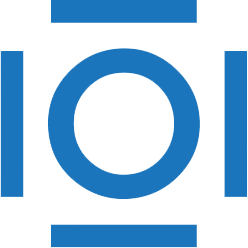
CITATIONS
Cited by 47 scholarly publications.
Feature extraction
Lithography
Data modeling
Machine learning
Lawrencium
Manufacturing
Detection and tracking algorithms