|
ACCESS THE FULL ARTICLE
No SPIE Account? Create one
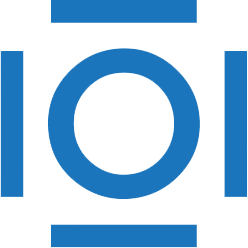
CITATIONS
Cited by 14 scholarly publications.
Image segmentation
Lithium
Signal to noise ratio
Optimization (mathematics)
Data modeling
Associative arrays
Hyperspectral imaging