Background: In datasets for hotspot detection in physical verification, data are predominantly composed of non-hotspot samples with only a small percentage of hotspot ones; this leads to the class imbalance problem, which usually hinders the performance of classifiers. Aim: We aim to enrich datasets by applying a data augmentation technique. Approach: We propose a data augmentation flow-based generative adversarial network (GAN) to generate high-resolution hotspot samples. Results: We evaluated our flow with the current state-of-the-art convolutional neural network hotspot classifier by comparison with conventional data augmentation techniques. Experimental results demonstrate that the accuracy improvement of our work can reach 3% at the same false alarm rate and the false alarm rate reduction can reach 5% at the same accuracy. Conclusions: Our study demonstrates that rational hotspot classification can improve the efficiency of data. It also highlights the potential of GAN to generate complicated layout patterns. |
ACCESS THE FULL ARTICLE
No SPIE Account? Create one
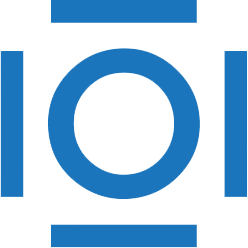
CITATIONS
Cited by 1 scholarly publication.
Gallium nitride
Data modeling
Performance modeling
Binary data
Machine learning
Computer programming
Convolution