The traditional numerical analysis in waveguide design can be time-consuming and inefficient. This is even more prominent in the THz region and with complex shapes and materials. As an alternative to overcome these drawbacks, we propose a machine learning (ML) approach to design porous-core photonic crystal fibers (PCFs) for the THz band. This method is based on an artificial neural network (ANN) model trained to predict key parameters such as the effective refractive index, effective area, dispersion, and loss values with accuracy and speed. In that sense, the network was trained to perform multiple-output regression of the above parameters. The training data for this model comes from numerical calculations that use the finite element method (FEM) to simulate and evaluate analytical expressions. Our results demonstrate the ML model’s ability to capture the complex and nonlinear relationships between the input and output parameters and accurately predict the behavior of the THz PCF. Moreover, the proposed model has an inference time of |
ACCESS THE FULL ARTICLE
No SPIE Account? Create one
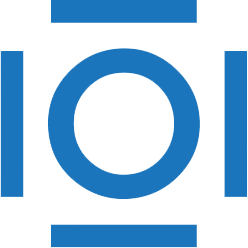
CITATIONS
Cited by 1 scholarly publication.
Terahertz radiation
Dispersion
Artificial neural networks
Waveguides
Data modeling
Porosity
Education and training