|
ACCESS THE FULL ARTICLE
No SPIE Account? Create one
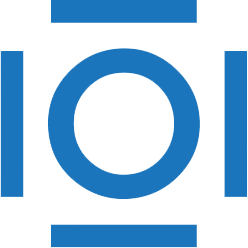
CITATIONS
Cited by 3 scholarly publications.
Calibration
Data modeling
Process modeling
Autoregressive models
Lithography
Photomasks
Monte Carlo methods